
This makes the task harder, since the neural network must now learn to generalize to more randomized environments. As the neural network gets better at the task and reaches a performance threshold, the amount of domain randomization is increased automatically. This frees us from having an accurate model of the real world, and enables the transfer of neural networks learned in simulation to be applied to the real world.ĪDR starts with a single, nonrandomized environment, wherein a neural network learns to solve Rubik’s Cube. To overcome this, we developed a new method called Automatic Domain Randomization (ADR), which endlessly generates progressively more difficult environments in simulation. Factors like friction, elasticity and dynamics are incredibly difficult to measure and model for objects as complex as Rubik’s Cubes or robotic hands and we found that domain randomization alone is not enough.
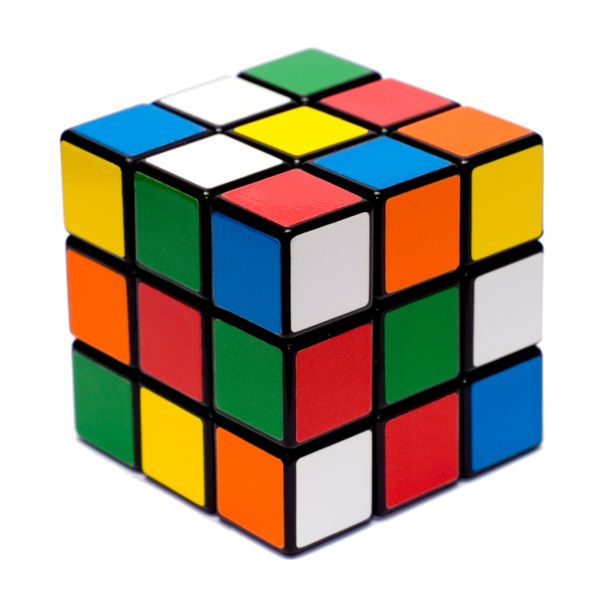
The biggest challenge we faced was to create environments in simulation diverse enough to capture the physics of the real world. Domain randomization enables networks trained solely in simulation to transfer to a real robot.ĭomain randomization exposes the neural network to many different variants of the same problem, in this case solving a Rubik’s Cube. We train neural networks to solve the Rubik’s Cube in simulation using reinforcement learning and Kociemba’s algorithm for picking the solution steps. Our robot still hasn't perfected its technique though, as it solves the Rubik’s Cube 60% of the time (and only 20% of the time for a maximally difficult scramble). Solving a Rubik’s Cube one-handed is a challenging task even for humans, and it takes children several years to gain the dexterity required to master it. This video plays at real-time and was not edited in any way. But as of July 2018, we could only manipulate a block on the robot. We solved the Rubik’s Cube in simulation in July 2017. We set this goal because we believe that successfully training such a robotic hand to do complex manipulation tasks lays the foundation for general-purpose robots.
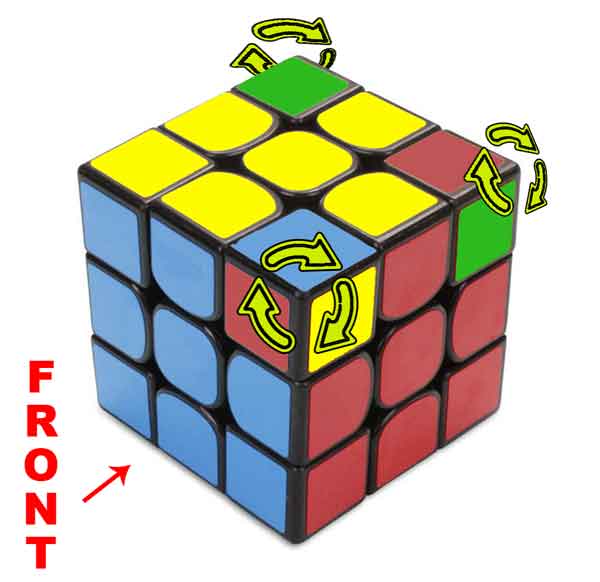
Since May 2017, we've been trying to train a human-like robotic hand to solve the Rubik’s Cube.
Solving runix cube software#
In particular, the hardware we use here is not new-the robot hand we use has been around for the last 15 years-but the software approach is. As an alternative, people have spent many decades trying to use general-purpose robotic hardware, but with limited success due to their high degrees of freedom.

For the past 60 years of robotics, hard tasks which humans accomplish with their fixed pair of hands have required designing a custom robot for each task. Human hands let us solve a wide variety of tasks.
